Scion data scientists working with remote sensing experts Indufor have developed a new commercial service to monitor forest establishment and indigenous forest fragments.
New Zealand will need to plant forests at unprecedented rates for decades to come if the country wishes to achieve its carbon neutrality goals and develop a renewable and sustainable bioeconomy. Successful establishment of newly planted trees is a critical factor in this. The most practical way to monitor establishment is using remote sensing techniques, but the current practice based on unmanned aerial vehicles is expensive and limited in scale.
Scion and Indufor are developing a quicker and more economic method that can be used over large areas.
Scion’s part in the work has been using deep learning to automate data monitoring with a high level of accuracy A neural network model is trained (via deep learning) to progressively extract higher-level features from the raw input. Rather like doing a jigsaw puzzle with a person in the picture, pixels that make up edge pieces are identified in an initial layer then the edge pieces are joined up in a subsequent layer, followed by a layer that learns large features, like a nose or an eye, and an additional layer will use these features to recognise the image contains a face, and so on.
The deep learning process automatically learns which image features are useful for the task at hand. This learning requires a high-quality training and validation dataset, often involving careful human image labelling. The training process is complete when the automatically learned model parameters are optimised in a way that the model gives the best possible performance on the unseen validation dataset.
Training sets are used by Scion to train the deep learning model for a specific problem. The model development started from locating and drawing a bounding box around areas that had been spot-sprayed after planting. However, this only works well with relatively homogeneously sized and shaped features – trees and forests tend to be irregular. The model was extended to combine object (sprayed spot) detection with a component that classifies each pixel in a bounding box. This enables sprayed spots and irregular forest fragments to be identified, mapped and delineated with polygons; data that can be used to evaluate seedling survival rate, while also gaining a clear picture of any indigenous forest pockets on site.
Indufor is using its customised aircraft-mounted sensor suite to obtain high resolution (5 cm) aerial imagery owned by aerial survey operator SKYVUW, however they had not previously used deep learning. The close collaboration with Scion has led to substantial investment in capability to turn the deep learning model into a commercial service. The commercial service can cover large areas economically. NZ Carbon Farming uses the service operationally and another forestry company is proposing to use it their new trial forest in Hawke’s Bay.
The combination of using deep learning with specialist remote sensing offers many advantages. Unlike earlier models, it needs much less data to train it. Large areas of afforested land can be covered quickly by a fixed-wing aircraft reducing the cost of data collection per unit area compared with UAV-based imagery. The data generation process also generates a 3D point cloud that could be potentially be used to characterise the terrain and biomass, height and density of any indigenous vegetation identified on a site.
The ability to automatically map indigenous forest data sets is the first step in understanding and improving the biodiversity value of afforested sites. Forest owners and managers are kaitiaki of indigenous forest fragments on the land they are responsible for. Knowing what is present allows them to manage the forest fragments to preserve and increase biodiversity, by, for example, integrating forest fragments, providing corridors for birds and other animals and encouraging natural revegetation. Setting aside significant natural areas is also a requirement for forest certification and will contribute to social licence to operate.
Well managed plantation and indigenous forests are vital to New Zealand’s economy, and long-term climate change response. This technology could play a role in ensuring those efforts are successful.
“Scion’s Geomatics team technical knowledge was instrumental in understanding the science behind the detection routines and giving us the confidence to invest in developing a scalable solution. Indufor’s collaboration with Scion within this fast-moving space has been an extremely positive experience and provides a fantastic example of applied science.” - Dr Pete Watt, Team Leader, Resource Monitoring & Climate Change, Indufor Asia Pacific Ltd
Funder: Strategic Science Investment Fund
Collaborators: Indufor and aerial survey operator SKYVUW
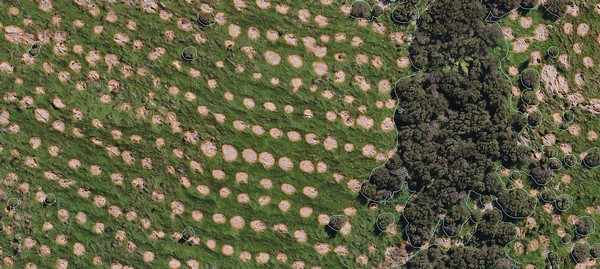