Forest Flows Technology
Integrating terrestrial measurements with novel remote-sensing technology
The success of Forest Flows is underpinned by the development of cutting-edge technological solutions.
- Novel datalogger equipment and wireless mesh network
- Innovative methods to measure soil moisture from the air
- A forest digital twin
- Unprecedented hydrology measurements
- A pipeline to handle big data volumes
- Machine learning and artificial intelligence tools for data analysis
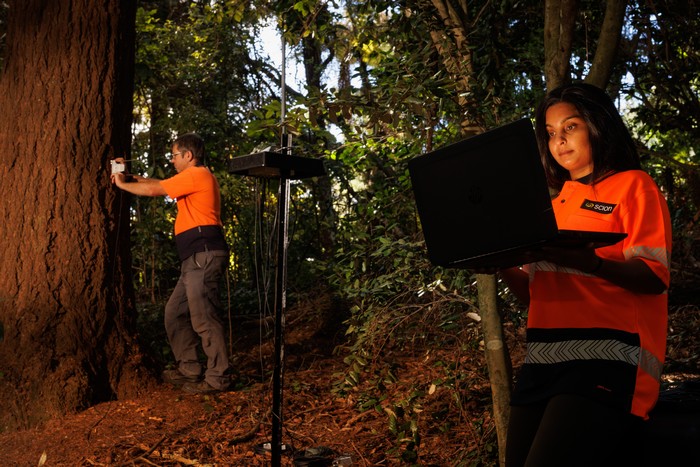
FlowLab Datalogger
Scion collaborated with the New Zealand technology Research and Design company INFACT Ltd, who developed a fit-for-purpose datalogger to work in challenging and remote forests.
- Low cost
- Battery powered
- Able to run different sensors
- Wireless meshed network to a central gateway
- Self-healing network
For each of the five primary research sites, 25 FlowLab dataloggers were deployed across the catchment, with each datalogger having four stem electronic smart dendrometers and four TEROS11 (Meter Group) soil moisture sensors.
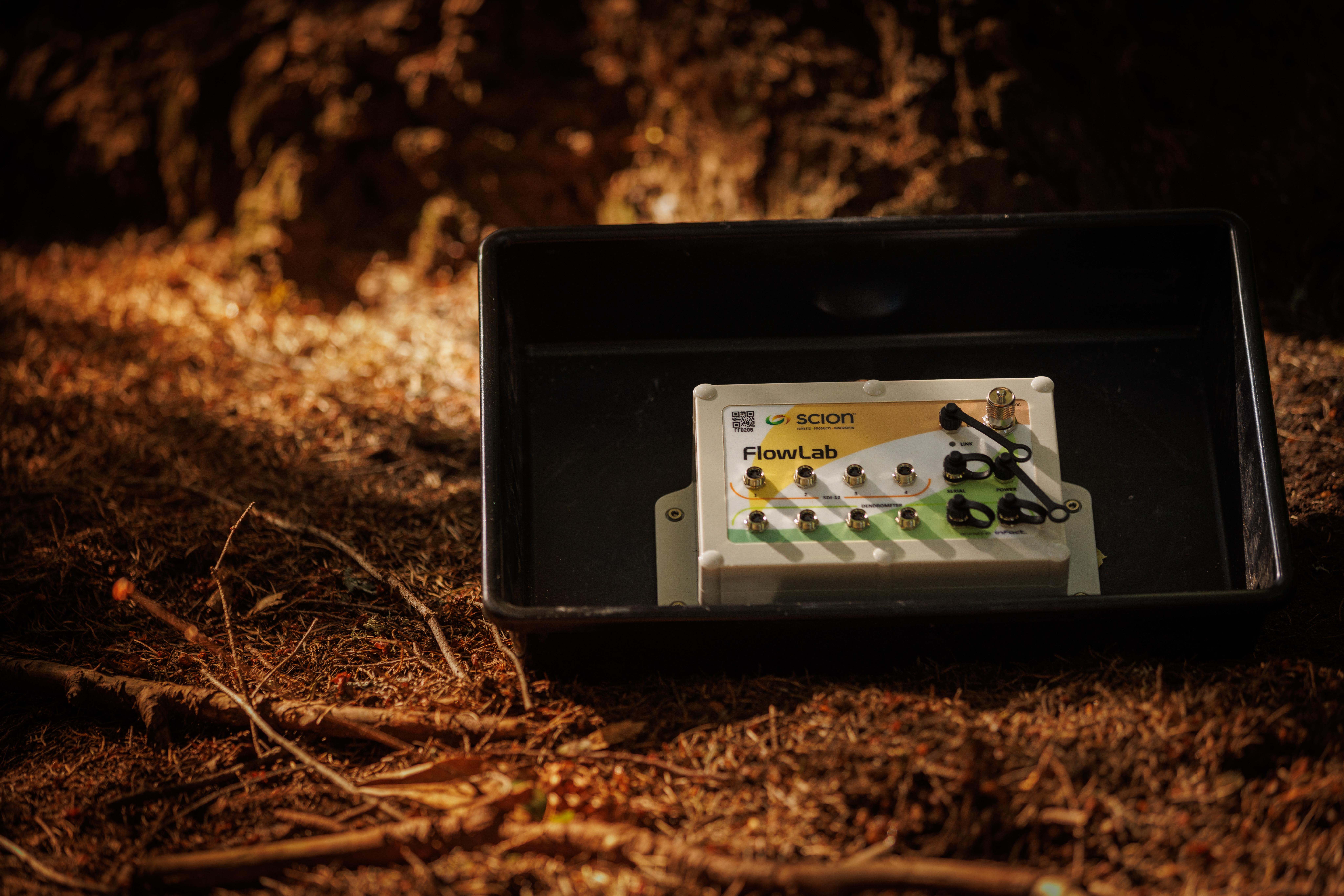
Measuring soil moisture from the air
SlimSAR, an aerial mounted L- and P-Band synthetic aperture radar (SAR), measured soil moisture from a plane, through the forest at a fine resolution to a depth of 1.5m. The University of Auckland and University of Southern California teams’ application of this technology is a world first, in dense canopy forests with steep and complex topography. SlimSAR as the potential to map soil properties and depth large areas and different land uses (e.g. agriculture and horticulture) quicker than current approaches and identify areas vulnerable to extreme weather events.
Integrating ground measurements
Forest Flows collected a range of ground measurements to support the sensor measurements including:
- Forest measurement plots
- Ground penetrating radar
- Soil and groundwater borehole cores
- Soil water retention curves
- Optical leaf area index measurements
- Surface LiDAR
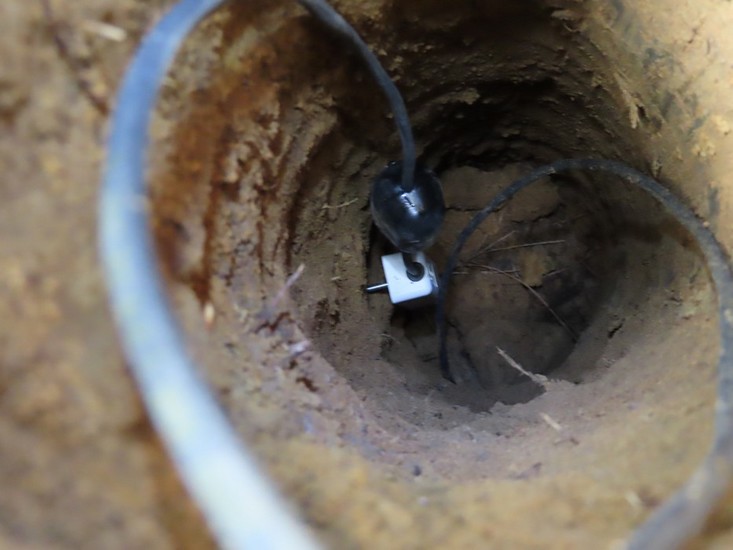
Kafka big data pipeline
To manage the 300,000+ daily observations, a big data pipeline was developed. A New Zealand first, Scion has developed the Forest Flows Kafka Big Data Pipeline that can seamlessly stream clean, summarise, and securely store big data arriving in near real-time straight from the forest from Scion and NIWA sensor networks. The cloud storage of terrestrial data made it simple for national and international collaboration.
Kafka enabled the fusion of data from intensive terrestrial site monitoring with remote sensing.
Analyse and Simulate
Forest Flows used a suite of statistical, empirical, modelling and AI methods. No one method was able to answer all the objectives of Forest Flows, rather using the strengths of each approach. Methods include:
Forest Flows Hydrological Model
Existing hydrology models are not-fit-for-purpose for forests because:
- They are based on agricultural soil models.
- They don't represent the different soil compositions, steeper topography, water drainage or soil systems found in New Zealand soils.
- Use static values (constants) to estimate forest water use (crop factors) or use a 'tipping bucket' model.
The Forest Flows hydrological model was created by Scion, NIWA, Moinhos de Vento Agroecology Centre and CSIRO links forests to the stream and provides accurate predictions of tree water use and stream water flux. This model is the combination of two models:
- CABALA-W: Upgrade of the well-regarded process-based forest model CABALA
- van Pauwels hillslope model
The Forest Flows Hydrological Model produces realistic predictions of streamflow, both for stream baseflow (between rainfall events), stormflow (during rainfall events) and the rate streamflow decreases after rain. CABALA-W provides accurate predictions of canopy interception, tree water use, and soil water movement and storage.
Deep Learning Approaches – Green AI
Forest Flows collaborated with the MBIE Time-Evolving Data Science/Artificial Intelligence for Advanced Open Environmental Science (TAIAO) data science programme led by the University of Waikato’s Artificial Intelligence Institute. This collaboration provides valuable synergies to both programmes with Forest Flows providing an example of complex environmental data streaming in near real-time.
A range of novel deep learning methods, considered Green AI, were developed from this collaboration:
- Deep Learning methods and efficacy of ML and data collection
- Predicting temporal/spatial variability of tree growth/water use within and between sites with a Deep Learning Neural Network
- Forecasting tree growth and water stress with Explainable AI
- Directly forecasting from data streaming from the forest
These methods are suitable for other ecosystems with complex environmental data.
Insights and Visualisation
3D Visualisation Tool
Scion collaborated with the NZ company Y5 to create a visualisation tool that demonstrates soil water movement through a catchment during a rainfall event. Using complex data collected from the programme it provides an easy-to-understand and interactive way to visualise water movement through the catchment from sensor measurements.